The Role of Big Data Analytics in Measuring Operational Efficiency and Resource Utilization
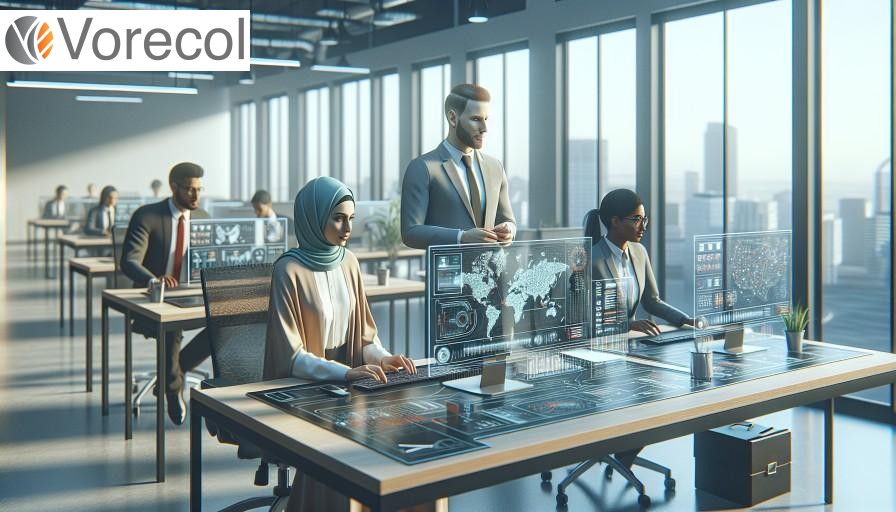
- 1. Understanding Big Data Analytics: A Primer
- 2. Key Metrics for Measuring Operational Efficiency
- 3. The Impact of Big Data on Resource Utilization
- 4. Case Studies: Successful Implementation of Big Data Analytics
- 5. Challenges in Integrating Big Data into Operational Processes
- 6. Future Trends in Big Data and Operational Efficiency
- 7. Conclusions: The Strategic Importance of Big Data Analytics in Business Operations
- Final Conclusions
1. Understanding Big Data Analytics: A Primer
In a world where the digital landscape is constantly evolving, the sheer volume of data generated daily is staggering. By 2025, it is estimated that the total amount of data created globally will reach 175 zettabytes, a number so large it’s hard to comprehend. This explosion of data has led businesses to invest heavily in big data analytics, with the global market projected to grow from $198 billion in 2020 to $684 billion by 2030, according to Fortune Business Insights. Companies like Netflix and Amazon have harnessed the power of big data to enhance their customer experience, utilizing predictive analytics to recommend shows and products alike, ultimately increasing consumer engagement and driving sales. In fact, 78% of organizations report that they analyze big data to gain insights that lead to more informed business decisions.
Imagine a local coffee shop that starts tracking customer preferences through a simple loyalty app. By analyzing this data, they identify that 60% of their customers prefer seasonal flavors, like pumpkin spice in fall or peppermint mocha in winter. Armed with this insight, the owner creates targeted marketing campaigns that result in a staggering 40% increase in sales during the holiday season. This scenario highlights how even small businesses can leverage big data to achieve remarkable results. With a staggering 79% of organizations believing they will be disrupted by digital transformation, the need to understand and implement big data analytics is not just an option but a necessity in today’s competitive marketplace.
2. Key Metrics for Measuring Operational Efficiency
In the bustling world of modern business, operational efficiency is the silent architect of success, shaping how companies allocate resources and deliver value. Consider the case of a leading manufacturer, which, after implementing a robust system for measuring its key metrics, reported a staggering 30% increase in productivity within just six months. Key performance indicators (KPIs) such as Overall Equipment Effectiveness (OEE) play a crucial role in this transformation. OEE not only gauges machine usage but also highlights areas where improvement is necessary; studies show that organizations that monitor OEE regularly can see efficiency gains of up to 25%. This underscores the importance of comprehensive metrics, serving as a lighthouse guiding businesses through operational storms.
Moreover, the importance of measuring cycle time cannot be overstated. A recent survey conducted by the Pulse of the Industry revealed that companies optimizing their cycle time improved their time-to-market by an astonishing 40%, thereby gaining a competitive edge in their respective fields. Additionally, measuring inventory turnover can reveal crucial insights into operational efficiency. With an average inventory turnover ratio of 6 to 8 for efficient retailers, businesses with higher ratios are seen to have a direct correlation with reduced holding costs and increased profitability. By embracing these key metrics, companies are not only enhancing their operational efficiency but also crafting compelling narratives of growth and innovation that speak to their stakeholders.
3. The Impact of Big Data on Resource Utilization
In the rapidly evolving landscape of business, the utilization of Big Data has become a game-changer for companies looking to optimize resource management. Imagine a manufacturing plant that, through the integration of Big Data analytics, identifies inefficiencies in its supply chain, reducing operational costs by up to 20%. According to a 2022 McKinsey report, companies that harness Big Data effectively can improve their productivity by 5-6%, leading to increased revenue margins and ultimately, a stronger market presence. This paradigm shift not only streamlines resources but also fosters a culture of innovation, where data-driven decision-making takes center stage, enabling firms to anticipate market trends and respond proactively.
Moreover, the impact of Big Data transcends manufacturing and seeps into various industries including healthcare, where it has revealed eye-opening statistics. A study by the American Journal of Managed Care highlighted that hospitals employing Big Data analytics have improved patient outcomes by 15% while simultaneously decreasing operational costs by nearly 25%. Picture a hospital that analyzes patient data to optimize staffing schedules, ensuring that resources are allocated where they are most needed, particularly during peak times. This transformative approach is indicative of a broader trend: as businesses grapple with the volume and complexity of data, those embracing Big Data are not just enhancing their resource utilization—they are redefining their entire operational framework, turning challenges into opportunities for growth and efficiency.
4. Case Studies: Successful Implementation of Big Data Analytics
In the bustling world of retail, Target’s implementation of big data analytics serves as a compelling case study. By harnessing customer purchasing data, the company successfully predicted a customer’s pregnancy based solely on their shopping patterns, leading to a staggering 50% increase in sales in expectant mother products. Their data scientists identified that pregnant women tended to buy unscented lotion and specific vitamins, allowing Target to personalize marketing efforts efficiently. This strategic move not only enhanced customer experience but also demonstrated a striking return on investment, as companies leveraging big data analytics see an average revenue increase of 8% and cost reductions of 10%, according to McKinsey.
In the healthcare realm, the use of big data analytics at Mount Sinai Health System transformed patient care and operational efficiency. By analyzing vast amounts of patient data, the hospital reduced readmission rates by 20%, saving an estimated $1 million in costs. Through predictive analytics and real-time data monitoring, patients at risk were identified, enabling preemptive interventions. The impact was profound; a study by Frost & Sullivan found that the healthcare sector could save up to $300 billion annually through effective big data practices. Mount Sinai not only improved patient outcomes but showcased how analytics could provide lifesaving insights and significant financial benefits, paving the way for other institutions to follow suit.
5. Challenges in Integrating Big Data into Operational Processes
Integrating big data into operational processes can feel like navigating a labyrinth for many organizations. According to a McKinsey report, companies that are equipped to harness big data can increase their operating margins by up to 60%, yet 70% of businesses still struggle to effectively capture and analyze this data. One striking example is Target, which famously used predictive analytics to anticipate customer needs, leading to a 20% increase in sales during a single promotional campaign. However, the success story also serves as a cautionary tale, highlighting the risks of data misinterpretation that resulted in privacy concerns and public backlash. This illustrates that while the potential rewards of big data are monumental, the integration process is fraught with challenges that can derail even the most promising initiatives.
As organizations grapple with these complexities, one critical challenge emerges: data silos. A recent survey conducted by Deloitte found that 56% of executives believe that siloed data hinders their ability to extract meaningful insights. Additionally, a report from Statista revealed that 41% of data-driven companies cite data quality issues as a major barrier to successful integration. Beyond technology, the human element plays a significant role; changing organizational culture to embrace data-driven decision-making can be as tough as any technical hurdle. Companies like Coca-Cola have invested not only in technology but also in training programs to instill a data-focused mindset among employees, reflecting the intricate dance between technology, culture, and strategy that defines the integration of big data into operational processes.
6. Future Trends in Big Data and Operational Efficiency
As companies move further into the digital age, the integration of big data analytics into operational strategies has become a game changer. For instance, a recent survey by McKinsey & Company found that organizations leveraging big data can enhance their operational efficiency by up to 30%. This transformative power is evident in industries such as manufacturing, where predictive analytics can foresee machinery failures, reducing downtime by as much as 50%. Additionally, businesses are investing heavily in big data technology, with IDC estimating worldwide spending on big data and analytics solutions to reach $274 billion by 2022, highlighting the essential role of data-driven decision-making in achieving financial and operational success.
Consider the story of Home Depot, a leading home improvement retailer. After adopting a big data strategy, the company was able to optimize its supply chain and inventory management, resulting in a 15% increase in operational efficiency within just one year. By analyzing customer purchasing patterns and seasonal trends, Home Depot cut excess inventory costs and ensured that popular products were always in stock. As firms navigate future trends, the emphasis on real-time data analytics and machine learning will only grow: Gartner predicts that by 2025, 70% of organizations will be using AI for operational decision-making, redefining productivity standards across industries. It's a future where data doesn't just inform strategy; it drives it.
7. Conclusions: The Strategic Importance of Big Data Analytics in Business Operations
In the rapidly evolving landscape of modern business, the integration of big data analytics has emerged as a critical differentiator among industry leaders. A McKinsey report found that companies that harness data-driven decision-making are 23 times more likely to acquire customers and 6 times more likely to retain them. This shift towards analytics not only enhances operational efficiency but also empowers companies to predict market trends and consumer behaviors. For instance, retail giant Walmart analyzes over 2.5 petabytes of data every hour, allowing them to adjust pricing strategies and inventory levels in real-time. The impact of these insights is profound, leading to increased sales and a stronger position in a fiercely competitive market.
Furthermore, a study by Bain & Company revealed that businesses leveraging big data can see productivity gains of up to 8-10%. This staggering percentage highlights the potential for increased efficiency and profitability through informed decision-making. Take the case of General Electric (GE), which utilizes the Industrial Internet of Things (IIoT) to analyze operational data from its jet engines and other machinery. By predicting maintenance needs, GE has reduced downtime significantly, saving billions in maintenance costs over time. These compelling statistics underline not only the strategic importance of big data analytics in refining business processes but also its essential role in redefining competitive dynamics across various sectors.
Final Conclusions
In conclusion, the integration of big data analytics into operational processes has transformed how organizations measure efficiency and resource utilization. By harnessing vast amounts of data, companies can uncover patterns and insights that were previously obscured, leading to more informed decision-making. This analytical approach not only allows organizations to optimize their operations but also fosters a culture of continuous improvement. In an increasingly competitive landscape, the ability to leverage big data effectively can differentiate leaders from laggards, driving sustainable growth and enhancing overall performance.
Moreover, as big data technologies continue to evolve, organizations must remain agile and adaptive to harness their full potential. The convergence of machine learning and artificial intelligence with big data analytics presents new opportunities for real-time monitoring and predictive insights, further enhancing operational efficiency. As businesses navigate the complexities of resource utilization, the strategic implementation of big data will be paramount. Ultimately, embracing these advanced analytical capabilities is not just a trend; it is a necessity for organizations aiming to thrive in today’s data-driven economy.
Publication Date: August 28, 2024
Author: Gestiso Editorial Team.
Note: This article was generated with the assistance of artificial intelligence, under the supervision and editing of our editorial team.
💡 Would you like to implement this in your company?
With our system you can apply these best practices automatically and professionally.
PsicoSmart - Psychometric Assessments
- ✓ 31 AI-powered psychometric tests
- ✓ Assess 285 competencies + 2500 technical exams
✓ No credit card ✓ 5-minute setup ✓ Support in English
💬 Leave your comment
Your opinion is important to us